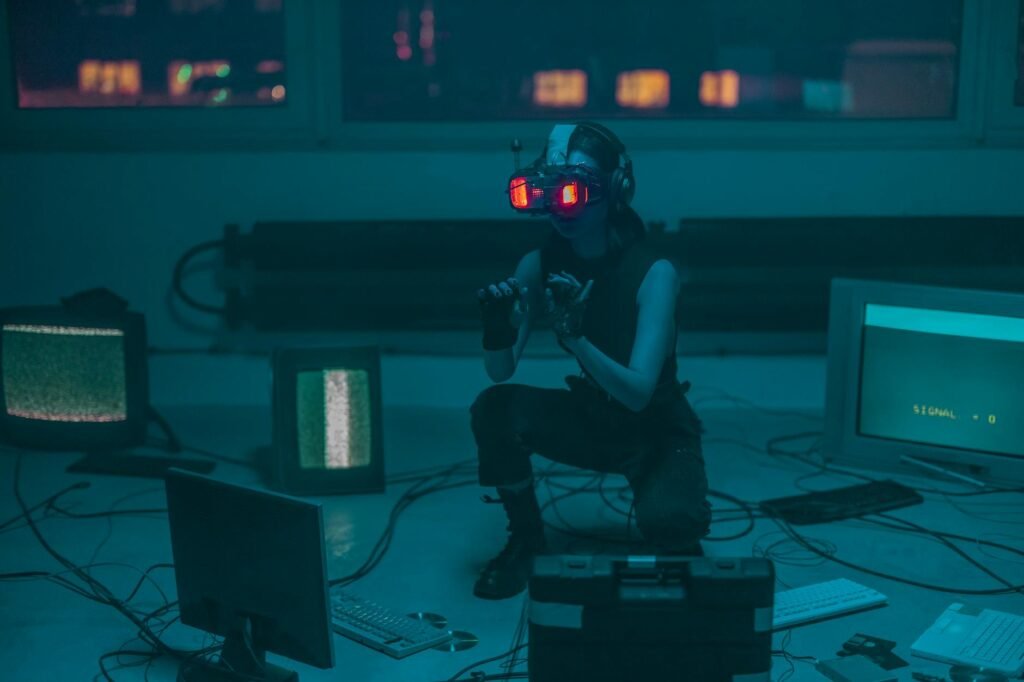
🤖 Imagine a world where AI systems generate content without any restrictions or oversight. From deepfake videos that could destabilize governments to AI-written code that could compromise critical infrastructure, the potential risks of uncontrolled artificial intelligence are becoming increasingly apparent. As these powerful tools become more accessible, the need for robust control mechanisms has never been more urgent.
why is controlling the output of generative ai systems important ?
The rapid advancement of generative AI has created a double-edged sword. While it offers unprecedented opportunities for innovation and creativity, it also presents significant challenges that could reshape our digital landscape – for better or worse. Without proper controls, we risk unleashing a torrent of misinformation, biased content, and potentially harmful outputs that could erode trust in digital systems and compromise societal stability.
In this comprehensive guide , we’ll explore the critical aspects of AI output control , from understanding current control mechanisms to implementing future-proof solutions. We’ll examine the technical approaches available today, discuss key stakeholder responsibilities, and outline the framework needed to ensure responsible AI development for tomorrow. 💡
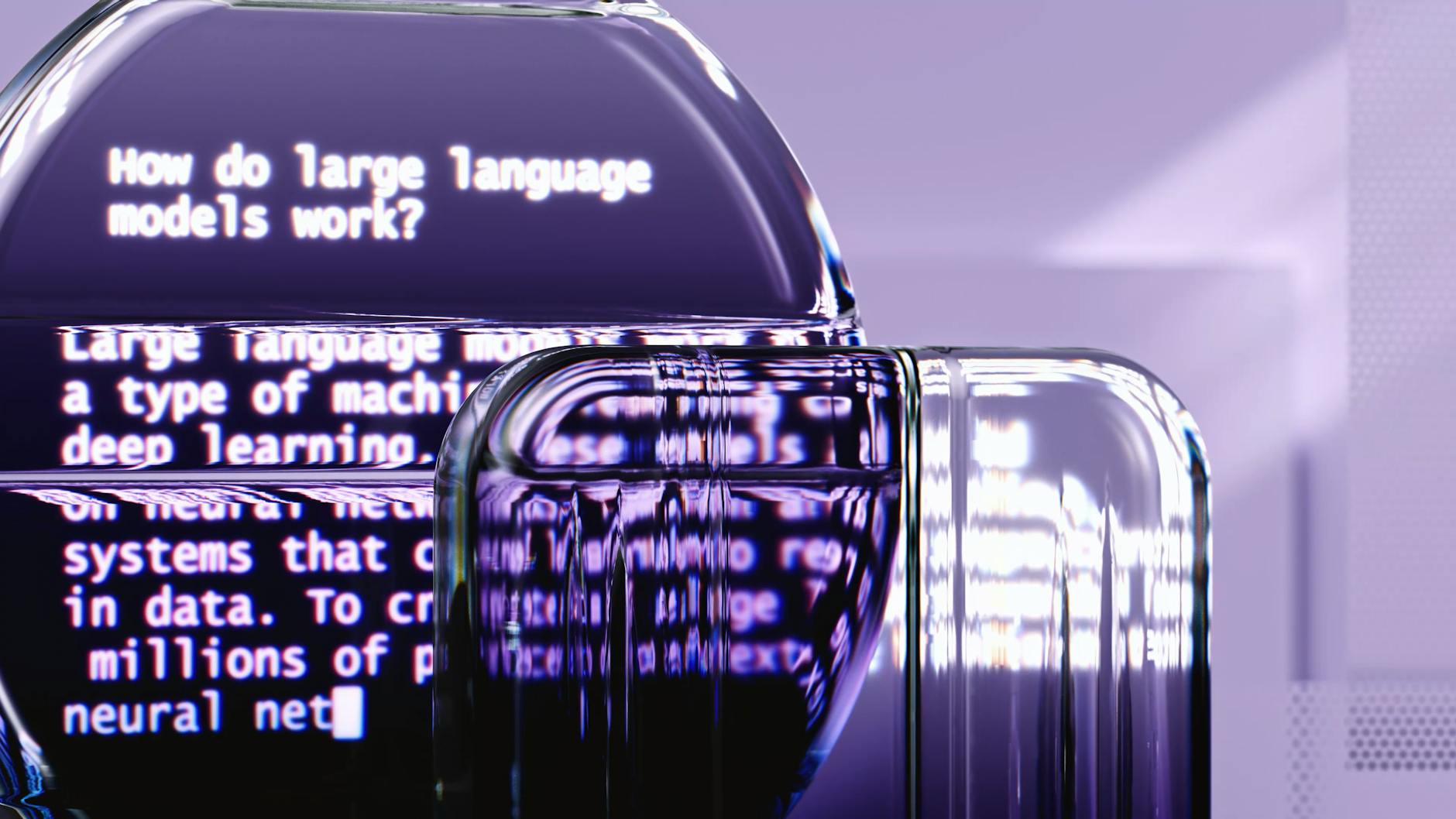
Understanding the Current State of AI Output Control
The Growing Influence of AI-Generated Content
AI-generated content has become increasingly prevalent across various sectors:
-
- Content Creation (text, images, videos)
-
- Software Development
-
- Business Analytics
-
- Customer Service
-
- Creative Industries
Sector | AI Adoption Rate | Primary Applications |
---|---|---|
Media | 76% | Content generation, editing |
Tech | 82% | Code generation, testing |
Service | 64% | Chatbots, support systems |
Creative | 58% | Design, art generation |
Current Challenges in Controlling AI Outputs
The rapid advancement of AI systems has introduced several control challenges:
-
- Accuracy and factual consistency
-
- Bias and fairness issues
-
- Content authenticity verification
-
- Output scalability management
-
- Quality control mechanisms
Real-world Examples of AI Output Mishaps
Recent incidents highlight the importance of proper AI output control :
-
- ChatGPT generating false legal citations in court documents
-
- AI art generators producing copyrighted material
-
- Language models creating misleading financial advice
-
- Image generators creating deepfakes of public figures
-
- Content generators spreading misinformation
These challenges demonstrate the critical need for robust control mechanisms. The potential risks of uncontrolled AI generation extend beyond mere inconvenience, potentially affecting social, economic, and political spheres. Now, let’s examine the specific risks that emerge when AI systems operate without proper controls.
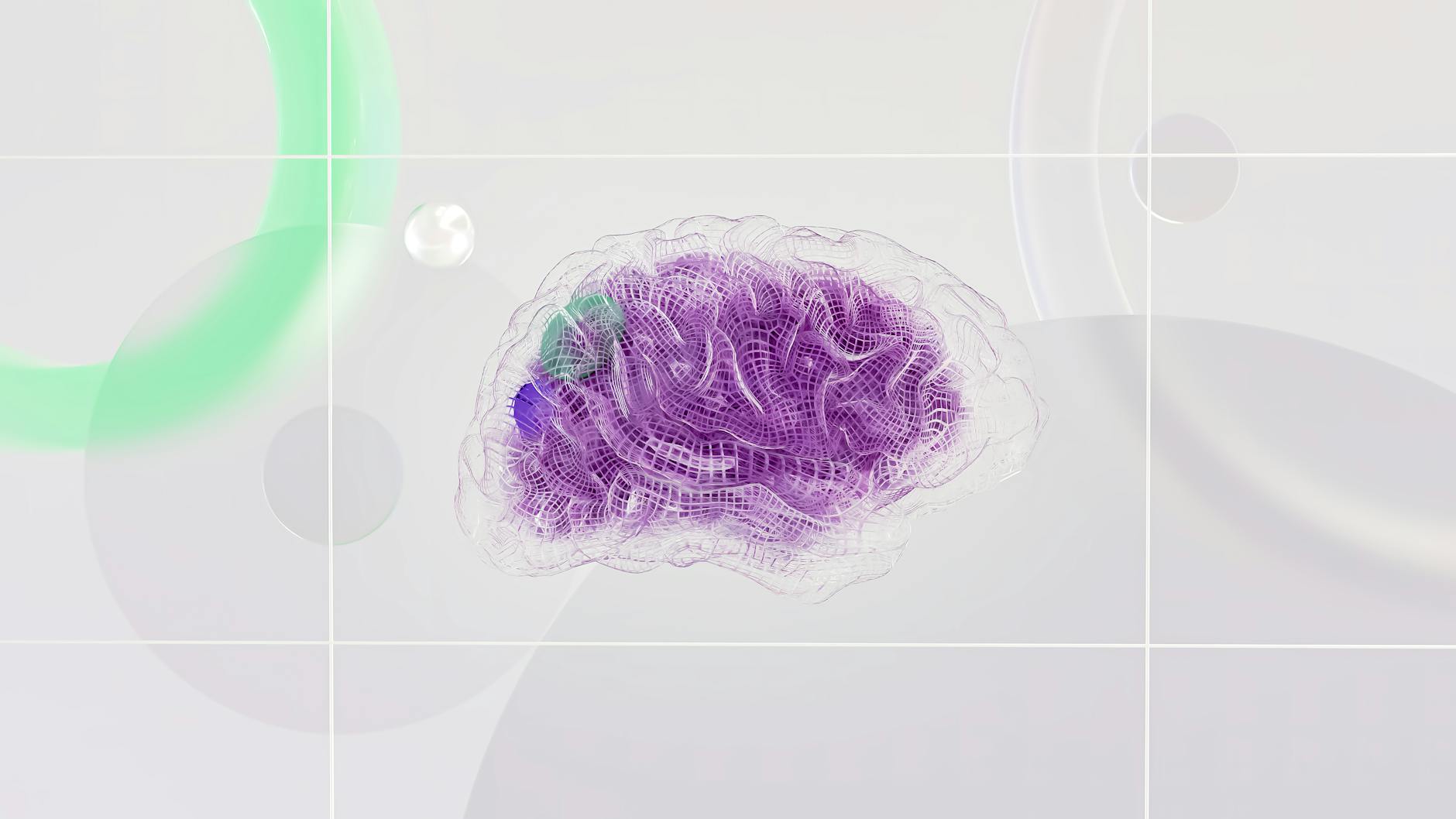
The Risks of Uncontrolled AI Generation
Misinformation and Fake Content Proliferation
AI systems can generate highly convincing fake content at unprecedented speeds, making it increasingly difficult to distinguish truth from fiction. Deepfakes, synthetic text, and manipulated media pose significant threats to :
-
- Information integrity
-
- Public discourse
-
- Democratic processes
-
- Brand reputation
-
- Individual privacy
Privacy and Security Concerns
The uncontrolled generation of AI content creates substantial vulnerabilities:
Risk Category | Potential Threats |
---|---|
Personal Data | Identity theft, Digital impersonation |
Corporate | Trade secret exposure, Social engineering |
National | Infrastructure attacks, Intelligence leaks |
Economic Implications
Unrestricted AI generation poses several economic challenges:
-
- Job displacement in creative industries
-
- Market manipulation through automated content
-
- Intellectual property violations
-
- Increased costs for content verification
-
- Disruption of traditional business models
Ethical Considerations
The ethical ramifications of uncontrolled AI generation extend beyond immediate practical concerns:
-
- Consent and ownership of training data
-
- Bias amplification in generated content
-
- Accountability for AI-generated harm
-
- Impact on human creativity and authenticity
-
- Psychological effects of synthetic relationships
The widespread availability of powerful AI generation tools without proper controls creates a complex web of interconnected risks. As we explore technical approaches to AI output control, it’s essential to understand how these various risk factors influence the development of effective control mechanisms.
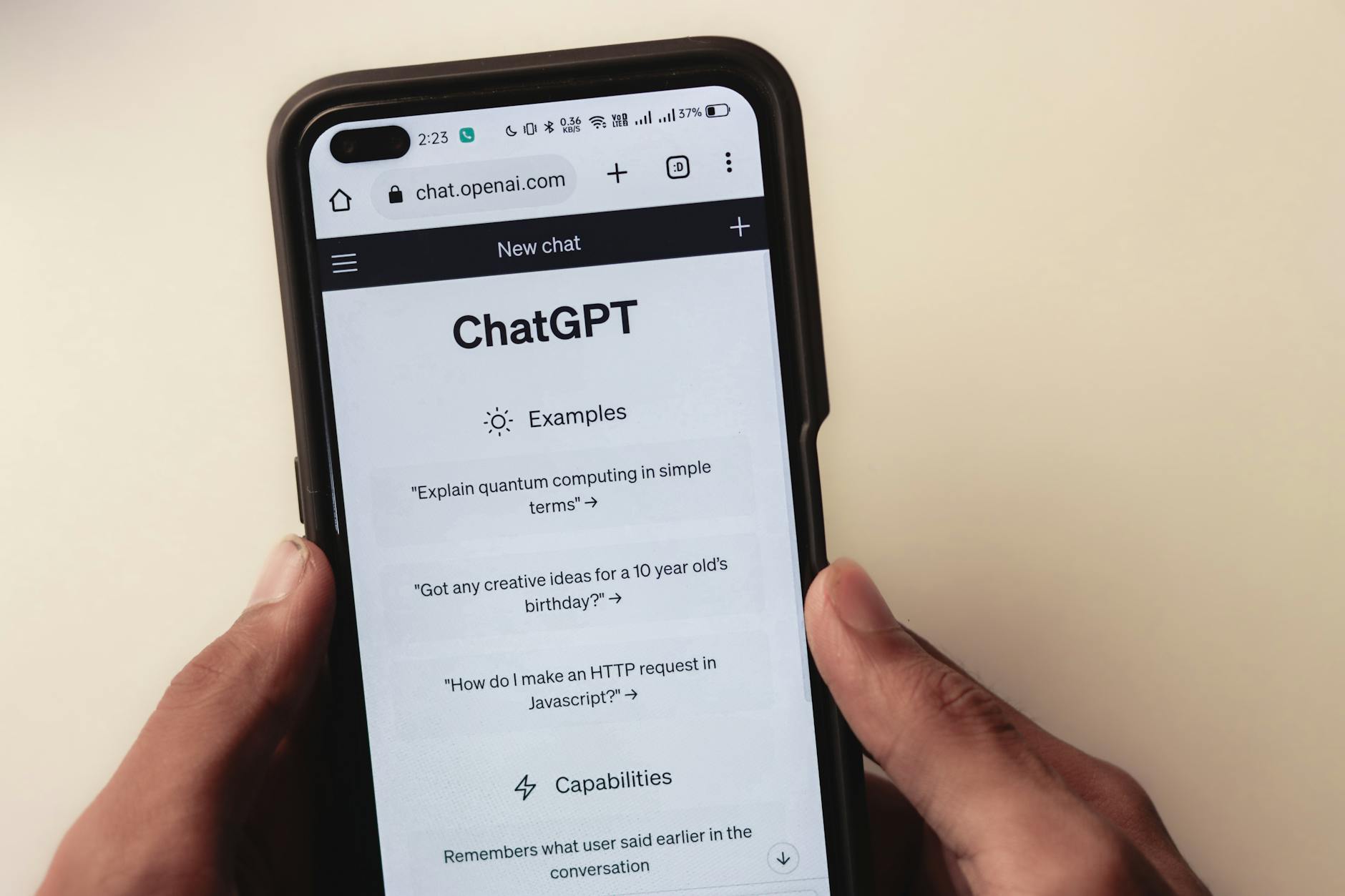
Technical Approaches to AI Output Control
Content Filtering Mechanisms
Content filtering serves as the first line of defense in controlling AI outputs. Modern filtering systems employ multi-layered approaches:
-
- Pattern matching algorithms
-
- Natural language processing filters
-
- Context-aware content screening
-
- Real-time toxicity detection
These mechanisms work together to create a robust filtering pipeline that screens content before it reaches end-users.
Output Validation Systems
Output validation ensures AI-generated content meets predefined quality and safety standards through:
Validation Layer | Purpose | Implementation |
---|---|---|
Syntax Check | Ensures proper format | Automated parsing |
Semantic Analysis | Verifies meaning | NLP algorithms |
Factual Verification | Confirms accuracy | Knowledge base comparison |
Safety Assessment | Identifies risks | Multi-model evaluation |
Human-in-the-Loop Oversight
Human oversight remains critical for maintaining control over AI outputs. Key components include:
-
- Expert review panels for complex decisions
-
- Real-time monitoring systems
-
- Feedback loops for continuous improvement
-
- Emergency override capabilities
These technical approaches work synergistically to create multiple checkpoints for AI-generated content. The combination of automated filtering, systematic validation, and human supervision provides a comprehensive framework for maintaining control over AI outputs.
With these technical controls in place, stakeholders can better understand their roles and responsibilities in maintaining safe AI systems.

Stakeholder Responsibilities
Developer Accountability
Developers hold primary responsibility for implementing robust control mechanisms within AI systems . They must:
-
- Design transparent algorithms with built-in safety checks
-
- Implement comprehensive testing protocols
-
- Document all control measures and potential risks
-
- Maintain regular update cycles for security patches
Platform Provider Obligations
Platform providers serve as gatekeepers and must ensure:
Responsibility | Implementation |
---|---|
Content Filtering | Real-time monitoring systems |
User Protection | Identity verification & access controls |
System Stability | Regular maintenance & updates |
Data Security | Encryption & privacy safeguards |
User Awareness and Education
Users require proper training and understanding through:
-
- Clear usage guidelines and documentation
-
- Regular updates on system capabilities and limitations
-
- Training modules on responsible AI usage
-
- Access to support resources
Regulatory Body Roles
Regulatory bodies must establish and enforce:
-
- Industry-wide standards for AI output control
-
- Compliance frameworks and audit procedures
-
- Penalty systems for violations
-
- International cooperation protocols
Corporate Governance Requirements
Organizations must implement:
-
- Clear AI usage policies
-
- Risk assessment procedures
-
- Regular compliance audits
-
- Stakeholder reporting mechanisms
With these responsibilities clearly defined, establishing effective control frameworks becomes more achievable. The success of AI output control depends heavily on how well each stakeholder fulfills their role in this ecosystem. Building a sustainable framework requires active participation from all these stakeholders working in concert.

Building a Sustainable Framework
Standards and Best Practices
-
- Regular security audits
-
- Output validation protocols
-
- Ethical guidelines compliance
-
- Data privacy standards
-
- Performance benchmarks
Implementation Guidelines
Phase | Key Activities | Success Metrics |
---|---|---|
Planning | Risk assessment, stakeholder alignment | Approved framework |
Development | Technical implementation, testing | Pass rate >95% |
Deployment | Staged rollout, monitoring | System stability |
Maintenance | Updates, optimization | Continuous compliance |
Monitoring and Evaluation Systems
A robust monitoring system must include:
-
- Real-time output tracking
-
- Accuracy measurements
-
- Bias detection algorithms
-
- Performance analytics
-
- User feedback integration
Continuous Improvement Protocols
To maintain effectiveness, organizations should:
-
- Implement quarterly framework reviews
-
- Establish feedback loops with end-users
-
- Update control mechanisms based on emerging threats
-
- Conduct regular staff training
-
- Document lessons learned and best practices
The framework must be adaptable to accommodate technological advances while maintaining strict control standards. Organizations should focus on creating scalable solutions that can evolve with AI capabilities. Regular assessments help identify potential vulnerabilities before they become significant issues.
Integration with existing IT infrastructure ensures seamless operation and reduces implementation barriers. Teams should prioritize automated monitoring tools to reduce manual oversight requirements while maintaining high security standards.
With this robust framework in place, organizations can focus on future-proofing their AI control systems to address emerging challenges and opportunities.

Future-Proofing AI Control Systems
Adaptability to Emerging Technologies
AI control systems must evolve alongside rapid technological advancements. Key adaptability features include:
-
- Modular architecture for easy updates
-
- Flexible integration points for new AI models
-
- Runtime configuration capabilities
-
- Plugin-based extension system
Scalability Considerations
Scaling AI control mechanisms requires careful planning across multiple dimensions:
Scaling Aspect | Requirements | Benefits |
---|---|---|
Vertical | High-performance computing resources | Handles complex AI models |
Horizontal | Distributed processing capability | Manages multiple AI instances |
Data | Efficient storage and retrieval systems | Supports growing training sets |
Cross-platform Compatibility
Modern AI control systems need to operate seamlessly across different environments:
-
- Cloud-native deployment options
-
- Containerized implementations
-
- Standard API protocols
-
- Platform-agnostic monitoring tools
The implementation of these future-proofing measures requires constant evaluation and adjustment. Control systems must maintain backward compatibility while incorporating support for emerging AI frameworks and methodologies. Organizations should establish regular review cycles to assess the effectiveness of their control mechanisms and identify areas requiring enhancement.
With the rapid pace of AI advancement, ensuring these systems remain robust and adaptable is crucial. Now, let’s examine how these various components work together to build a comprehensive framework for AI governance.
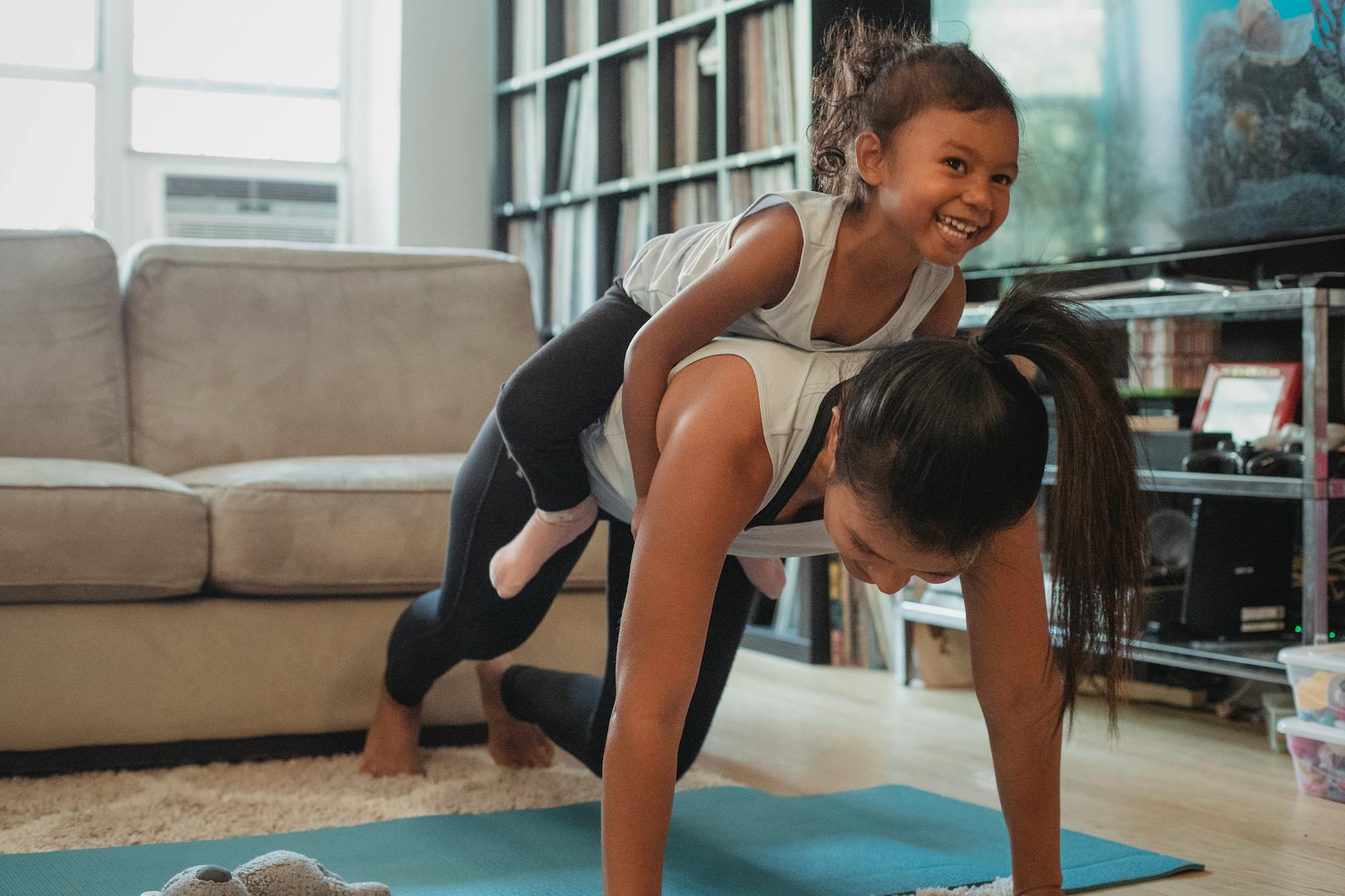
Effective control over generative AI outputs stands as a cornerstone of responsible technological advancement. From implementing robust technical safeguards to establishing clear stakeholder responsibilities, the steps we take today will shape how AI systems impact our society tomorrow. The development of sustainable frameworks and control mechanisms ensures that AI remains a tool for progress while minimizing potential risks and misuse.
The path forward requires collaboration between technologists, policymakers, and the public to create adaptable control systems that evolve with AI capabilities. By prioritizing output control now, we can harness the tremendous potential of generative AI while maintaining the ethical boundaries and safety measures necessary for a secure digital future. The time to act is now – let’s work together to shape AI development that serves humanity’s best interests.